We regularly hear from clients who want to invest in a generative AI proof of concept POC or roadmap but are unsure about the tech stack or the team they need. How can you ensure it progresses to production to drive observable impact? Thatʼs what weʼll walk you through in this article, including insights from our webinar From POC to Production: Navigating GenAI Implementation in Life Sciences.
Choosing the right roadmap
In our experience, generative AI implementation in life sciences is a spectrum. The first stage is proof of concept. This is followed by scaled generations, then optimized generations, and the final stage is a fully embedded roadmap.
Starting small with a proof of concept is a sensible first step, but we often see clients choose too narrow a use case. While it can prove the approach, it can be challenging to know how to scale to different applications.
On the other hand, going all in on a generative AI roadmap without a POC exposes you to greater risk in terms of ongoing technology fluctuations, in addition to other macro factors such as regulation.
When we reviewed the success factors for generative AI projects weʼve been involved in, we realized thereʼs a goal-oriented sweet spot on the spectrum. Depending on your technology and team readiness, this can mean one of two goals:
- Driving observable impact through a meta use case, such as medical affairs
- Driving better actions through multiple meta use cases across multiple user types
Setting one of these goals as the North Star for your roadmap helps minimize
your risk while ensuring your proof of concept leads to scalable output. It alsoprovides the necessary context for the next element of generative AI implementation: your technology.
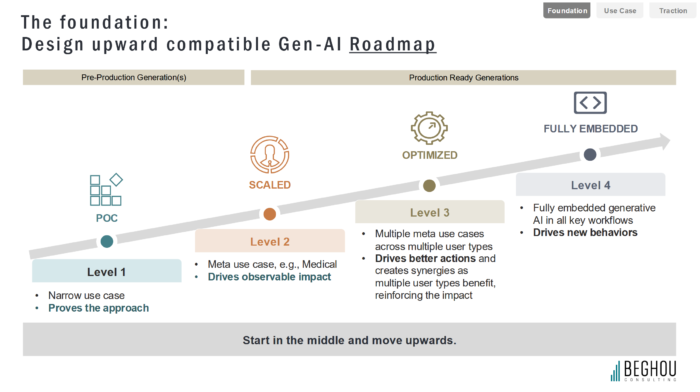
Setting up the tech stack for your roadmap
One of the benefits of generative AI is that itʼs innately suited to “building upwardsˮ, a design philosophy that enables scale and flexibility in your tech stack. It starts with finding your “Goldilocksˮ entry point—not too basic, not too complex—then growing and evolving it upwards toward your vision.
This is also spectrum that increases in complexity and resource intensiveness. The stages are:
- Large language model (LLM) – pre-trained, out-of-the-box technology
- Prompt engineering – customize the AIʼs instructions
- Retrieval-augmented generation (RAG) – combine LLM with existing data
- Fine-tuning – refine an existing model
- Pretraining – train a model from scratch
While there are use cases for all of these stages in life sciences, in terms of moving from POC to production and maximizing ROI, you can solve most with the middle stages (prompt engineering and RAG). Rohit Gupta, Partner at Beghou Consulting states, “You may not even need a fine tune model. [For commercial operations and medical insights], if you have a well defined client- workflow and good prompt engineering, that solves 80/90% of all use cases we have.ˮ
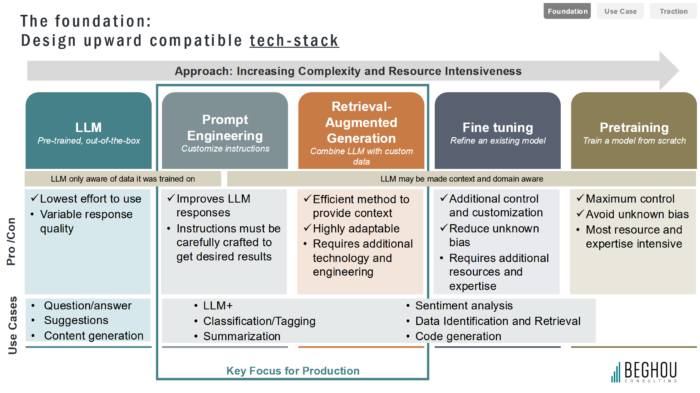
Employing the right skillsets for your tech stack
While itʼs true that you wonʼt get far without the right engineers and programmers, thereʼs also the persistent challenge of pulling through crucial business and human context in any generative AI POC.
Rohit shares: “There is this either explicit or implied issue that people feel a little insecure about generative AI. Some jobs may be lost, so a lot of change management aspects come into the picture.ˮ
So who do you need on your team? Here are some roles you could consider from the start:
- Data Engineer or Data Analytics: Your technical expert who understands the integration needs of your existing tech stack and can drive implementation.
- Business Analyst: Your domain knowledge expert who contributes business insights and nuances to your potential use cases or roadmap.
- Sponsor and change management lead: Your change management champion who can plan and lead communications that help prevent concerns around jobs becoming barriers to adoption.
Your initiative will, of course, need more than three people to develop. But securing these three roles will enhance its relevance for solving business problems and improve internal perceptions of the project. In turn, these factors will increase the chances of a successful transition from POC to production and maximize the projectʼs ROI.
Access the webinar slides
If youʼre looking for more insights like these, weʼd recommend reviewing the slides from our webinar “From POC to Production: Navigating GenAI Implementation in Life Sciencesˮ. Youʼll discover more best practices for generative AI implementation from two Beghou Partners and one of our clients.