case study:
90% accuracy achieved for categorizing unstructured medical affairs data using a scalable, automated AI solution
Pharma company shifts to automated processing and reporting of CRM insights, gaining real-time outcomes while saving hours of manual effort per week
A pharma company struggled to achieve timely real-world insights from the vast amount of information contained in the unstructured call notes from field medical affairs reps stored in its CRM. The manual process of reading and categorizing the reports consumed considerable time and resulted in errors.
To optimize this process and gain more real-time insights, the company partnered with Beghou Consulting to adopt a natural language processing (NLP) approach that would scale with its needs and reduce the required resources.
AI solution
implementation timeline
Challenge: Extracting timely stakeholder insights
As the first competitor to the company’s lead product entered the market, the leadership team desired better insights into the real-world treatment landscape so it could differentiate itself from the competition.
The company recognized that the rich information collected from the field medical affairs reps’ during their interactions with healthcare professionals provided the insights they needed. However, although the reps recorded detailed notes in the company’s CRM, the leadership team was unable to extract insights in a timely manner due to the labor-intensive manual review process.
Issues with the review process included:
- Errors and biases in the categorization/tagging that resulted in missed information when it was aggregated.
- Manual review of the 1000s of notes again if a new signal emerged (e.g., a safety concern) that required a new tag.
- Considerable time, including more than 20 hours per month of administrative time for manual categorization, which was expected to increase over time.
- Delay in actionable insights, which were delivered only on a quarterly basis, limiting a timely response to the changing landscape.
Approach: Scalable, customized AI solution suited to the company’s needs
The Beghou team, including data scientists, medical analytics experts, and executive leadership (SMEs), leveraged our expertise to quickly implement a feasible NLP-driven AI solution to deliver the company’s vision of automated, reliable, timely insight tagging and more granular categorization and reporting. While this project was launched prior to the advent of GenAI, this technology could now be layered on to accelerate and enhance insight generation.
At Beghou, we base our customer interactions on a collaborative approach to ensure the end product meets the specific organizational needs and gain stakeholder buy-in from the very beginning.
For this project, this meant that the company’s clinical and medical teams, especially the regional directors, had multiple feedback opportunities during the iterative development process, including:
- Initial stakeholder interviews to align on the tags
- Review and input on the draft ontologies created by the Beghou team
- Review of additional tags identified by the NLP that were not in the original list
- Pilot testing of the system to incorporate changes before the full launch
- Review of the classification into the desired categories of “insight,” “intel,” and “neither” to ensure accuracy
- Feedback on the reporting and dashboards
Key business questions that were considered:
Are the medical notes insight or intel, or do they hold any actionable value (neither)?
Does this insight apply to another function (e.g., market access)?
What is the insight trend per classification over a given time?
Is the insight linked to a specific clinical trial?
Is there competitive intelligence within the insight?
How many insights are collected per insight type, product, healthcare professional, healthcare organization, key opinion leader, or territory?
The cross-functional collaboration ensured that the tags, ontologies, and outputs in the final product provided the specific insights the company needed, when they needed them.
The Beghou Customer Relationship and
Engagement Advantage
Traditional CRM platforms are complex, rigid, and difficult to use.
If you’re a modern, agile, and fast-moving team, you need an intelligent, flexible platform that can keep up with you.
Beghou CRE is designed to support your needs today and adapt seamlessly to the demands of tomorrow.
Example entries in the notes | Tags assigned by the system | Notes category |
---|---|---|
“Asset 1 has the highest safety and tolerability for XXX indication. However, there are issues with YYY.” | Asset, Efficacy & Safety, Indication
| Insight
|
“Asset 2 is likely going to produce better clinical results than comp 1. It will be interesting to assess which regime will have the best efficacy profile for NSCLC patients and how it will impact corresponding access restrictions.” | Asset, Clinical Biomarker, Competitor, Efficacy & Safety, Indication (NSCLC), Access
| Insight and intel
|
“Asset 1 might not be a strong drug for XXX indication, Asset 2 hepatotoxicity is not an issue and has a similar clinical profile as Asset 3”
| Asset (1, 2, and 3), Efficacy & Safety, Indication, Side Effects | Insight |
Example dashboards custom created for the
company’s needs
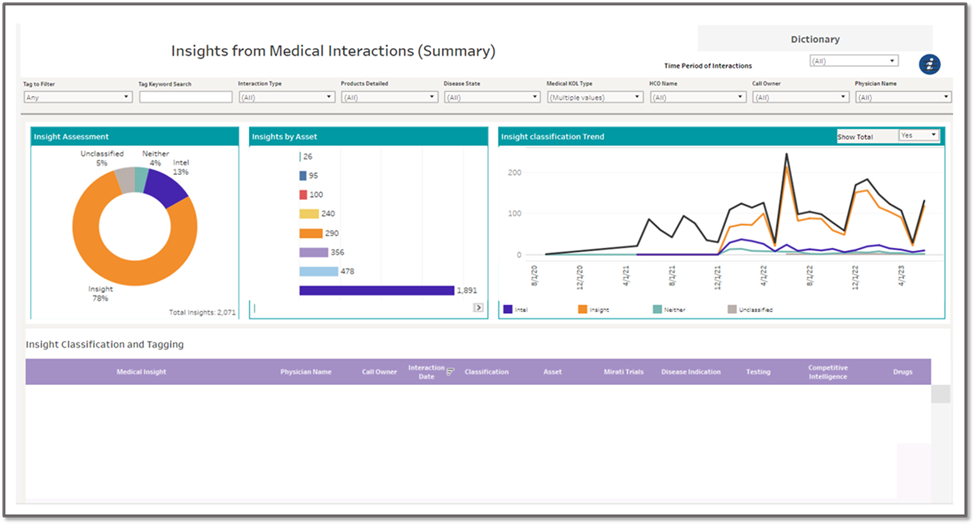
The system design also allowed for continuous progress, so we could meet the client where they were during the project and allow for future expansion as the market changed, such as adding tags to capture new types of information needed.
Simplified workflow for the NLP approach to tag, classify, and visualize notes
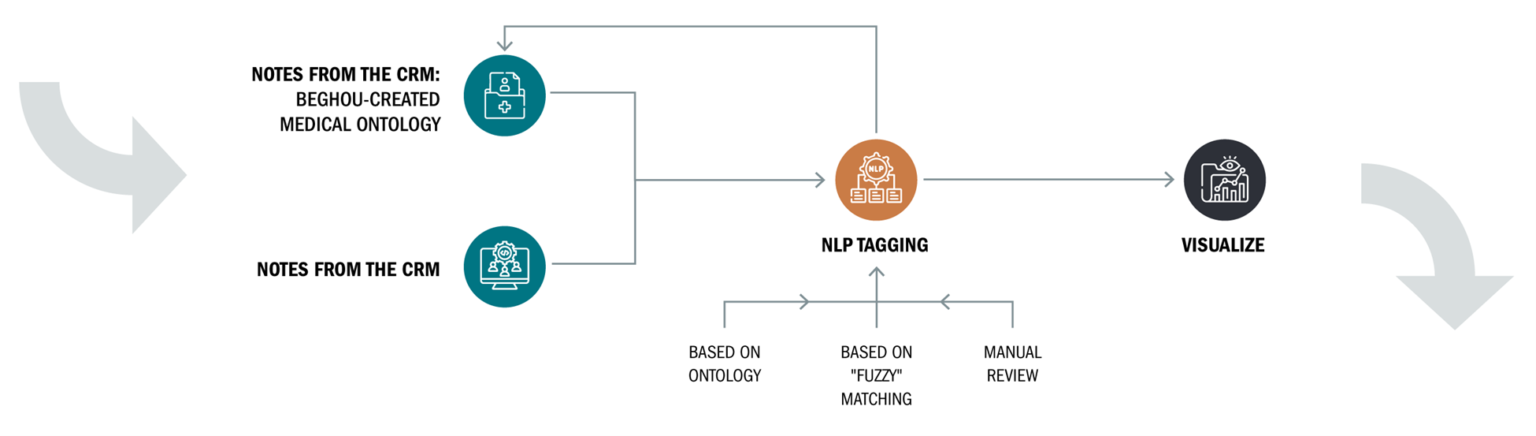
Additional planned improvements included sentiment analysis to better understand changes in perceptions of the assets over time; semantic searching to allow for different names for the same drug or clinical trial names, for example; and Q&A functionality using conversational AI (after the advent of GenAI) that would have allowed user-entered queries of the content. However, the company was acquired before the next phase of the project commenced.
Results: Launch success and market share
The NLP-based solution went live only 2.5 months after the initial list of tags was created. Once in production, it eliminated the time spent by the field medical affairs team, improved the accuracy of the findings, has the ability to grow with the company’s needs, and provides near real-time insights that could be used by the leadership team and the field medical affairs reps to plan their interactions with healthcare professionals.
Outcome | Beghou Approach | Direct results |
---|---|---|
Comprehensive classifications across multiple categories
| Collaborated with the company’s medical and clinical experts to create robust medical ontologies and training data to appropriately classify notes across various groupings (Insights, Intel, No action) |
|
Predictable actionability
| Iteratively validate and refine the process based on existing reports |
|
Highly configurable, scalable solution | Designed the model to be easily configured and able to integrate new data sources |
|
Built a feedback loop using periodic inputs
|
| |
Near real-time outcomes |
Configured the data pipelines and model triggers to classify the insights as soon as the CRM data were refreshed
|
|
User-friendly interface | Packaged the results and outputs into a self-service dashboard |
|
Driving success through partnership and change management
Another critical factor for a successful project like this one is organizational buy-in for what could be seen as disruptive technology, as well as changes to the existing processes. This was especially true for this company, as this was its first AI implementation. However, all sides approached this project as a partnership, and the company’s leadership and project team trusted the expertise of
Beghou’s team to not only implement the technology but also guide effective change management, which needs to start from project onset.
Some important considerations in this project were:
- Working with the med affairs team to incorporate their input and feedback about how the solution will fit into their workflows, the outputs that are provided, and how it can be improved.
- Incorporating a human feedback loop into the NLP and machine learning processes to reduce the perception of the solution as a “black box.”
- Early system demonstration in a pilot phase not only for system refinement but also to initiate excitement about its capabilities.
With the faster access to real-world insights, the company is able to better tailor its interaction strategies, identify trending topics, understand its customers’ concerns, and refine evidence planning in a rapidly changing environment — ultimately enhancing patient care and treatment outcomes based on inputs from treating physicians.
Learn more about Beghou’s medical affairs solutions and proven capabilities for leveraging both GenAI and NLP to optimize tagging and predictions even further by watching our webinar “From POC to Production. Navigating GenAI Implementation in Life Sciences.”