Biotech company enables faster, more accurate medical insights with AI
Challenge:
Solution:

Fit-for-purpose AI
solution that scales

Real-time insights vs.
manual quarterly reports

~90% accuracy of call
note categorization

Significant manual time
saved per week
Issues with the review process included:
- Errors and biases in the categorization/tagging that resulted in missed information when it was aggregated
- Manual review of the 1000s of notes again if a new signal emerged (e.g., a safety concern) that required a new tag
- Significant resource sink as analysts were tied up with manual categorization of notes for hours on end, which was expected to increase over time
- Delay in actionable insights, which were delivered only on a quarterly basis, limiting a timely response to the changing landscape
For this project, this meant that the company’s clinical and medical teams, especially the regional directors, had multiple feedback opportunities during the iterative development process, including:
- Initial stakeholder interviews to align on the tags
- Review and input on the draft ontologies created by the Beghou team
- Review of additional tags identified by the NLP that were not in the original list
- Pilot testing of the system to incorporate changes before the full launch
- Review of the classification into the desired categories of “insight,” “intel,” and “neither” to ensure accuracy
- Feedback on the reporting and dashboards
- Are the medical notes insight or intel, or do they hold any actionable value?
- Does this insight apply to another function (e.g., market access)?
- What is the insight trend per classification over a given time?
- Is the insight linked to a specific clinical trial?
- Is there competitive intelligence within the insight?
- How many insights are collected per insight type, product, healthcare professional, healthcare organization, key opinion leader, or territory?
Example entries in the notes | Tags assigned by the system | Notes category |
---|---|---|
“Asset 1 has the highest safety and tolerability for XXX indication. However, there are issues with YYY.” | Asset, Efficacy & Safety, Indication | Insight |
“Asset 2 is likely going to produce better clinical results than comp 1. It will be interesting to assess which regime will have the best efficacy profile for patients and how it will impact corresponding access restrictions.” | Asset, Clinical Biomarker, Competitor, Efficacy & Safety, Indication, Access | Insight and intel |
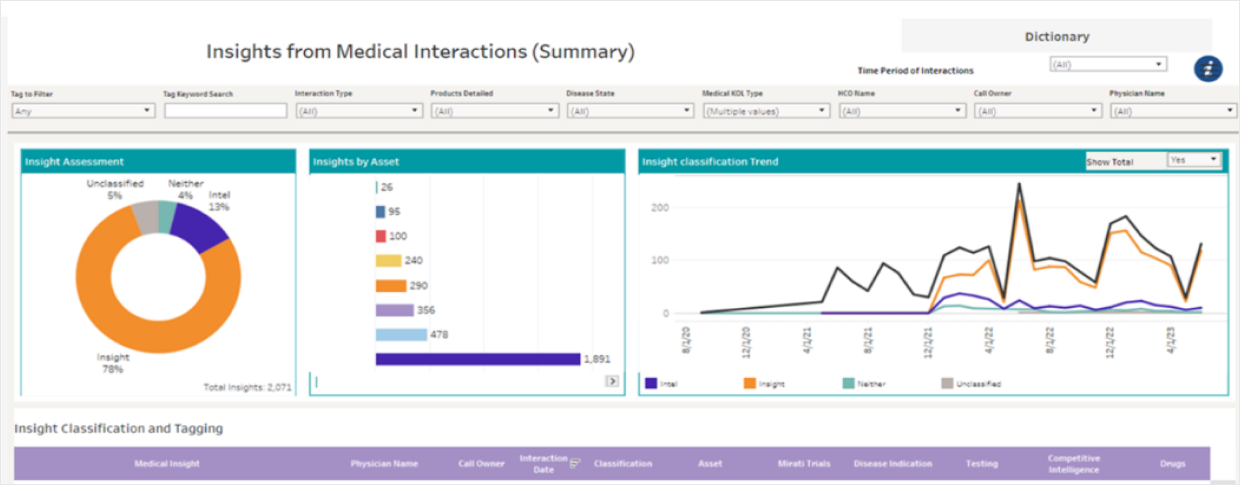
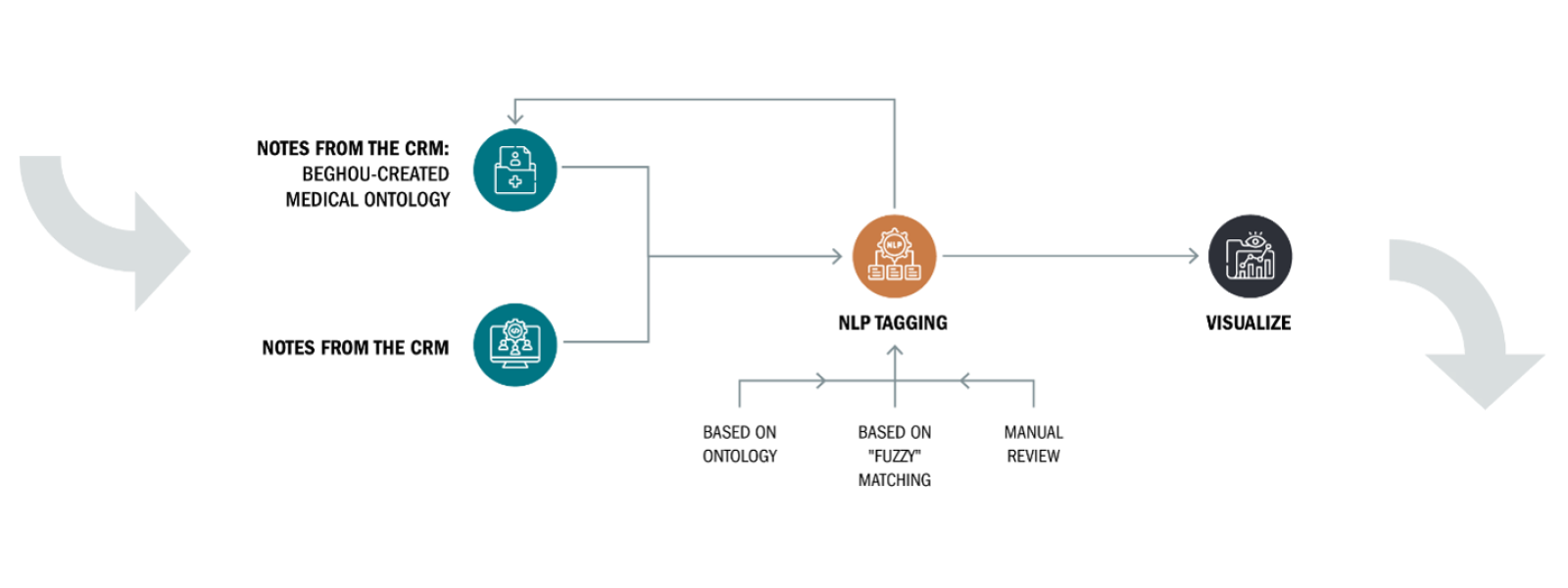
The NLP-based solution went live only 2.5 months after the initial list of tags was created. Once in production, it eliminated the time spent by the field medical affairs team, improved the accuracy of the findings, has the ability to grow with the company’s needs, and provides near real-time insights that could be used by the leadership team and the field medical affairs reps to plan their interactions with healthcare professionals.
Comprehensive classifications across multiple categories | Predictable actionability | Near real-time outcomes | User-friendly interface |
---|---|---|---|
Beghou approach Collaborated with the company’s medical and clinical experts to create robust medical ontologies and training data to appropriately classify notes across various groupings (Insights, Intel, No action) | Direct results
|
Beghou approach
Direct results
- The resulting classifications accurately and reliably reflected the content.
- The use of “fuzzy” matching accounted for different spelling and abbreviations, for example.
- The resulting classifications accurately and reliably reflected the content.
- The use of “fuzzy” matching accounted for different spelling and abbreviations, for example.
Beghou approach
Direct results
- High prediction accuracies (~90%) were achieved for categorizing the feedback into the groupings (Insights, Intel, No action).
- Intel or insight from relevant actions could be passed to the medical and clinical teams to plan follow-up activities with the stakeholders.
- High prediction accuracies (~90%) were achieved for categorizing the feedback into the groupings (Insights, Intel, No action).
- Intel or insight from relevant actions could be passed to the medical and clinical teams to plan follow-up activities with the stakeholders.
Beghou approach
Direct results
- The model can adapt as medical strategies change or a new signal is identified.
- The list of tags grew from 5-6 used during the manual process to ~40 tags in the automated process, and this can expand further, allowing for greater granularity in categorization and reporting.
Beghou approach
Direct results
- The model can be continuously improved based on SMEs’ classifications or insight assessments.
- Using machine learning, the system can also learn from new inputs over time and benefits from having a human expert in the feedback loop.
- The model can adapt as medical strategies change or a new signal is identified.
- The list of tags grew from 5-6 used during the manual process to ~40 tags in the automated process, and this can expand further, allowing for greater granularity in categorization and reporting.
- The model can be continuously improved based on SMEs’ classifications or insight assessments.
- Using machine learning, the system can also learn from new inputs over time and benefits from having a human expert in the feedback loop.
Beghou approach
Direct results
- The delay in insights with the manual process was significantly reduced.
- Processing of the notes occurred automatically every couple of hours, instead of weekly or less often.
- The delay in insights with the manual process was significantly reduced.
- Processing of the notes occurred automatically every couple of hours, instead of weekly or less often.
Beghou approach
Direct results
- Users can “slice and dice” the information at different levels of granularity to quickly read the call notes and plan subsequent follow-ups.
- Users can “slice and dice” the information at different levels of granularity to quickly read the call notes and plan subsequent follow-ups.
Some key considerations in this project were:
- Continual stakeholder collaboration: The importance of early collaboration with the med affairs stakeholders for their input and feedback on how the solution will fit into their workflows, the outputs that are provided, and how it can be improved.
- Human feedback loop: Incorporating a human feedback loop into the NLP and machine learning processes to reduce the perception of the solution as a “black box.”
- Early demos and stakeholder excitement: Early system demonstration in a pilot phase not only for system refinement but also to initiate excitement about its capabilities.
With the faster access to real-world insights, the company is able to better tailor its interaction strategies, identify trending topics, understand its customers’ concerns, and refine evidence planning in a rapidly changing environment — ultimately enhancing patient care and treatment outcomes based on inputs from treating physicians.
Learn more about strategies and case studies on how life sciences companies can use AI to drive business impact in our recent webinar “From POC to Production. Navigating GenAI Implementation in Life Sciences.”
Explore Similar Case Studies
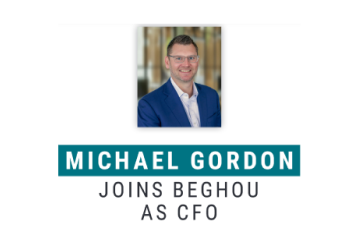
Michael Gordon joins Beghou
as Chief Financial Officer
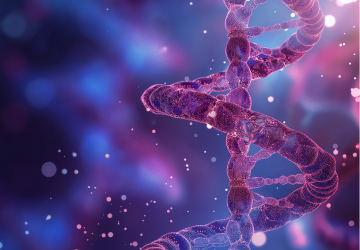